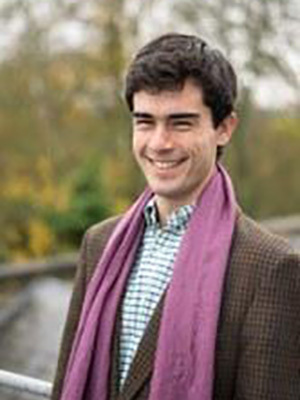
Dr André Neto-Bradley
At the DAFNI Conference 2022, Dr André Neto-Bradley introduced EnergyFlex, a microsimulation model that uses public data to synthetise a representative housing stock for any local authority, and allows users to estimate energy efficiency and characteristics of the housing stock in the area.
The model has been run using DAFNI and the outputs can be used to locally tailor and target energy efficiency and decarbonisation efforts. EnergyFlex was developed through a collaboration between the University of Cambridge, the University of Leeds, the Alan Turing Institute and DAFNI.
André explains:
With EnergyFlex we started out wanting to use as much public data as possible so that anyone could use the model without privacy concerns or GDPR issues, hence using synthetic populations of houses and synthetic households that live in them and that are representative of a local area.
We then use energy modelling to estimate some energy efficiency and energy performance characteristics of those houses. As we have representative socioeconomic characteristics of the household and the technical energy performance characteristics of the building, we can carry out some rather intricate policy and retrofit solution simulations, like a sandbox, to look at different solutions and see what the impact could be for that local authority.
We often get asked about the EPCs – the Energy Performance Certificates – and if you’ve bought, rented or sold a home since 2008 you should have seen one of these. It tells you how efficient your home is and tells you a little bit about the construction of the house, its energy performance and where improvements could be made
So why can’t we just use EPCs for our work? They’ve only been required since 2008 and they aren’t always that accurate, humans are doing the evaluations and there are approximations made; EPCs also have no socioeconomic context. In addition, EPCs are not anonymous so there would be privacy concerns if you wanted to carry out scenario simulation with this kind of data.
EnergyFlex has three key blocks:
- The population synthesis set where University of Leeds colleagues took the lead using their SPENSER model
- Two energy characteristic estimation steps:
- The first is the energy intensity modelling where we sample the energy intensity of a property based on the age and type of house.
- The second is the Bayesian calibration step which uses a sample of case study data to calibrate our energy consumption estimates and infer some of the building fabric allowing us to evaluate retrofit solutions.
In many cases real data on the housing stock and the households living in them does not exist or has not been collected to the degree required. There are also research ethics questions around how you could share research based on real household data, plus limits on what you could and couldn’t report in terms of disclosing sensitive information, so a synthetic housing stock and synthetic populations that are representative allow you to have all the data you need to optimise things for your local authority and share it without constraints.
How DAFNI helps researchers
DAFNI has provided us with a useful cloud-based platform for scheduling model runs and crunching numbers for the whole country. DAFNI is also useful for stakeholder engagement– it can be difficult to produce customised model outputs to respond to every stakeholder’s specific interests. DAFNI allows us to more easily respond to stakeholder’s questions by packaging up the model on DAFNI and allowing stakeholders and collaborators to run EnergyFlex for their area of interest without needing to be a coding expert. This enables us to share a user friendly workflow with other researchers, people working in local government and other stakeholders interested in housing. The interested parties can specify an input parameter such as the local authority they’re interested in (e.g. Leeds) and they can run the model and get the output data themselves.
DAFNI shares data and models in a way that’s easy for other people to access. On DAFNI models can be put out into the open so that you can integrate these with other outputs and inputs feeding into a variety of models, not just your own. DAFNI also allows researchers to help avoid duplication and to build on the work of others.
Use cases
Using EnergyFlex to look at trends across the whole country.
This map [insert graphic] shows the energy intensity of new build homes in England – those built since 2000. We found quite a significant correlation between house prices, disposable incomes and energy intensity and this can be useful in terms of identifying spatial inequality in risk of fuel poverty, identifying areas that require more support. We can also take this a step further and look within local authorities and look at each housing type and each age of housing to see how efficient homes of different types (e.g. 1930s Terraces vs 1970s Flats) in that local authority are. For example, in Leeds, a lot of the oldest homes are quite efficient compared to comparable homes in neighbouring and less urbanised local authorities and there’s a whole range of reasons why that may be. It may be that there’s a higher proportion of retrofits already, or that these homes are built differently. EnergyFlex is very much a rough calculation rather than a final decision-making tool but it allows for that initial high level view that can highlight the need for further action.
Simulating retrofit solutions
For the second use case we zoom in much further and look at simulating retrofit solutions. We worked with the London Borough of Haringey who supplied us with extra data to allow us to do that final calibration step and to estimate some of the building performance characteristics beyond just the energy intensity. Haringey is interesting because it’s almost entirely made up of flats and most of them were built before 2000.
Say we’re interested in inefficient heating systems, we could look at replacing boilers with air source heat pumps, it’s very topical.
Using EnergyFlex we can estimate the likely efficiencies of the heating systems in these different age bands of flats in Haringey and in this particular example [screenshot] we find that some of the most inefficient boilers are to be found in the 1930s to 1970s flats and 1970s to 2000s flats. That helps us to have an overview of types of houses to be targeted but we can also have a look at who’s living in the houses. In the 1970s to 2000s flats we see the highest proportion of households in Haringey in lower socio economic groups and we see that a lot of those 1970s -2000s flats are social rentals. Interestingly, the 1930s -1970s flats are mostly private rentals. So what does that mean when we’re looking at the big picture and simulating our policy?
Let’s say we are interested in replacing those inefficient boilers with heat pumps. The higher prevalence of inefficient boilers in the 1930s -2000s flats would be a good place to start, it’s low hanging fruit. But the households in the 1970s-2000s flats in lower socio economic groups may need financial support if the household was made to bear the cost of the improvements. Similarly for the 1930s-1970s flats as they are mainly private rentals we would probably have to find some way of incentivising or forcing private landlords to upgrade their heating.
Do get in touch if you’re interested in finding out more or in using EnergyFlex on DAFNI.
Contact: Marion Samler, Partnership Manager, DAFNI: Data & Analytics Facility for National Infrastructure Marion.samler@stfc.ac.uk.
Dr André Neto-Bradley, Research Associate, University of Cambridge
André is an engineer and data scientist, and has been a Research Associate at the Energy Efficient Cities initiative (EECi) since completing a PhD on modelling energy inequalities in urban India at University of Cambridge. His research interests focus on understanding and modelling the role of socio- cultural context in urban residential energy, as well as the use of data science approaches to characterise barriers and inequality in energy access and decarbonisation – leveraging publicly available data to support local decision making. He has recently started working as a Senior Energy Advisor at the Department for Business, Energy, and Industrial Strategy.
Interested in collaborating with DAFNI?
If you would be interested in using DAFNI, would like to access Data on DAFNI or involve us in a new project, we would like to hear from you. Please complete your details on the contact form using the link and we will be in contact with you by email.